Best Practices for Sentiment Analysis
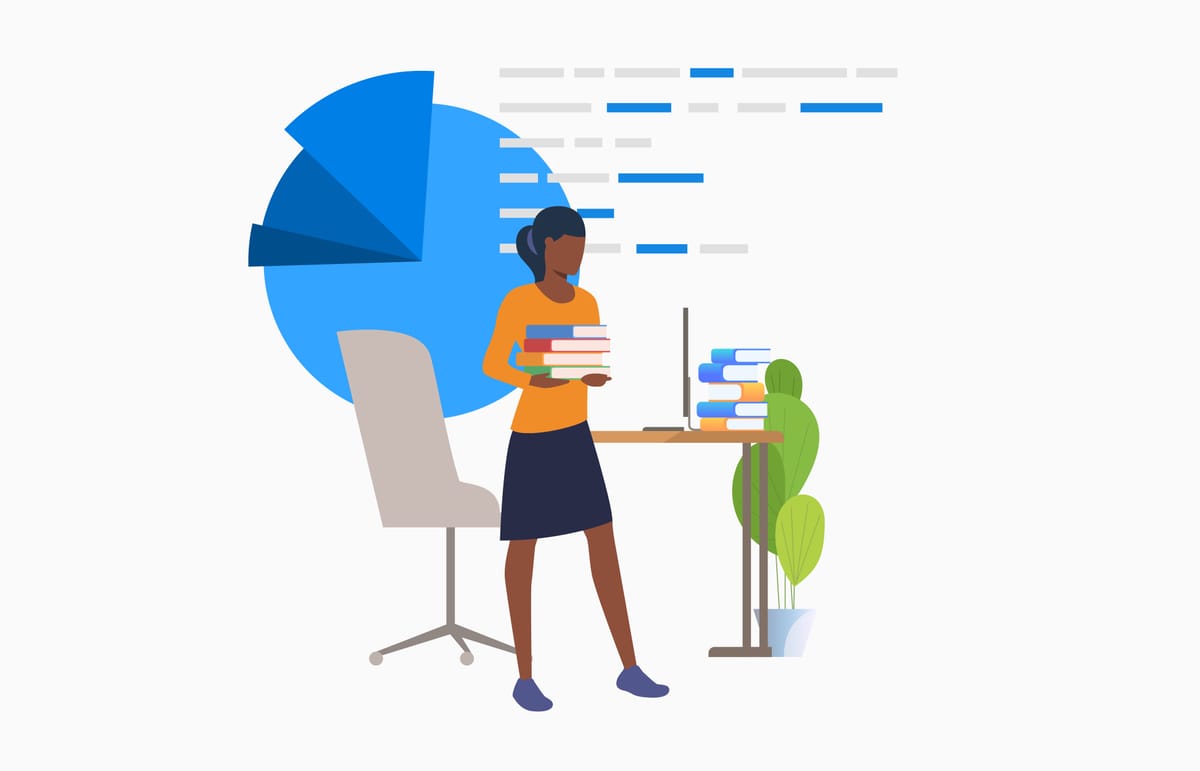
Understanding consumer sentiment is critical in any industry where brands and customer experiences reign. And in the era of big data, new techniques are empowering companies to accurately sense consumer attitudes, emotions and opinions at unprecedented scale.
Sentiment analysis is an advanced application of artificial intelligence and computational linguistics that mines subjective signals in text, social media, transcripts, ratings, and other human data. Essentially, sentiment analysis discerns opinions, feelings, judgments or attitudes which can then be quantified and analyzed.
Leading organizations leverage sentiment analysis to uncover powerful insights that drive marketing campaigns, product development, brand reputation management and beyond. But not all sentiment analysis programs achieve the same caliber of insight. Here are best practices to maximize accuracy and impact:
Frame Clear Business Objectives
Before investing in sentiment analysis capabilities, establish clear goals for what business challenges you hope to address or decisions you aim to enable. Common applications include:
- Understanding drivers of customer satisfaction/NPS to improve experiences
- Early detection of brand perception issues or crises based on social listening
- Monitoring opinion trends for targeting campaigns or positioning
- Gauging reactions and feature appeal during new product/service development
- Optimizing content marketing strategies and themes for maximum resonance
Well-defined objectives are crucial for focusing sentiment analysis accurately on the right data types and domains.
Identify Relevant Data Sources
With objectives mapped, the next step is sourcing appropriate data to feed into the analysis. Relevant sources typically include:
- Customer social media comments (forums, review sites, etc.)
- Contact center call transcripts, emails, and chat logs
- Open-ended feedback and survey responses
- Traditional media coverage and online press articles
- User-generated content including blogs, video transcripts, etc.
Data quality and representativeness are important factors to consider to drive valid insights. Proven sampling and statistical techniques should be applied as needed.