The billion-dollar race for AI supremacy
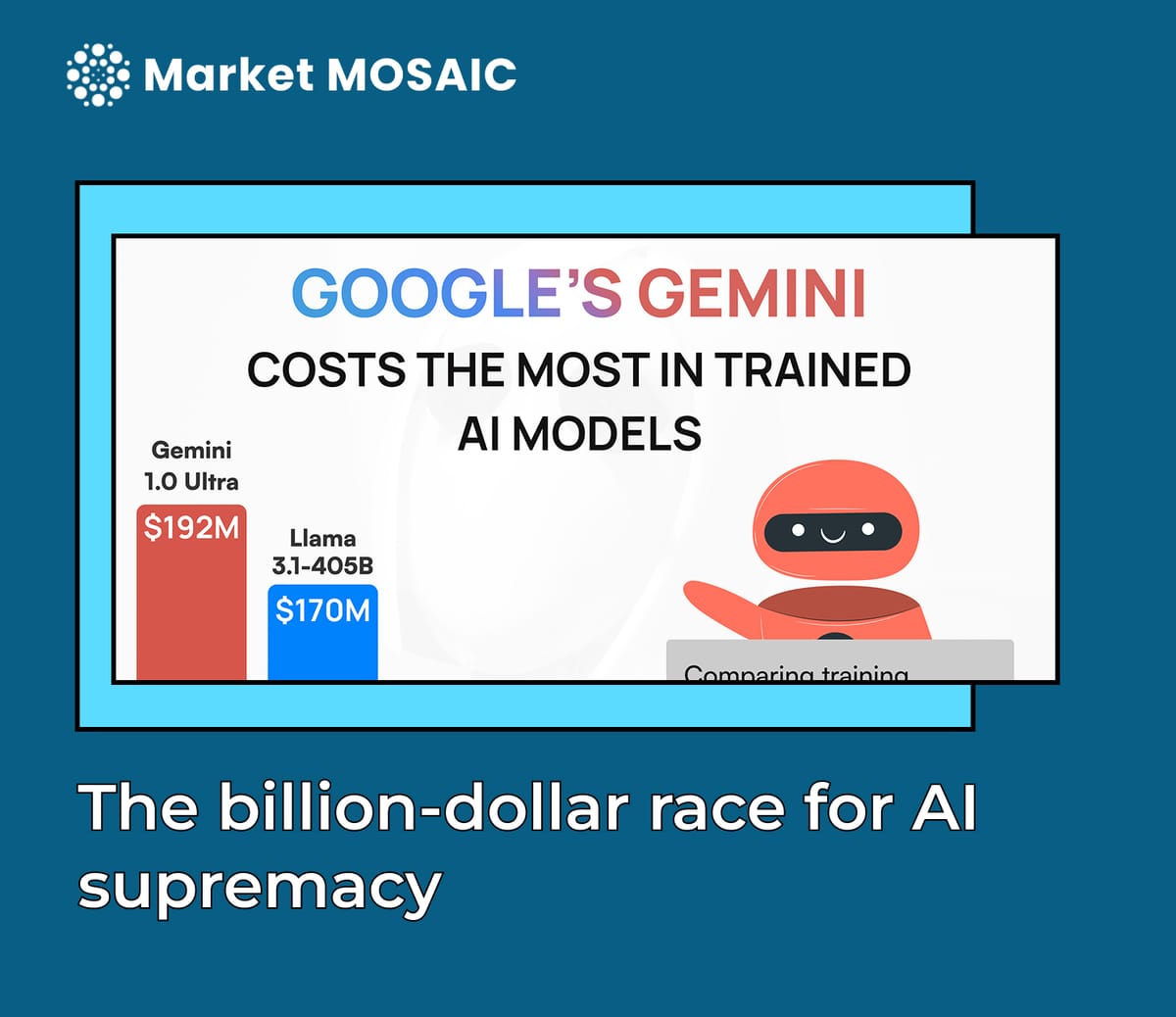
Unpacking the financial, strategic, and market implications of training large-scale AI models
In recent years, the cost of training large-scale AI models has surged, signaling a dramatic shift in the AI landscape. Google's Gemini 1.0 Ultra model cost $192 million to develop, reflecting the increasing financial barriers to entry. This investment in AI models is no longer limited to technological innovation; it’s a key factor in strategic business decisions across industries.
The economics of modern AI: where the money go
Analyzing Gemini’s $192 million training expense reveals how resources are distributed. Nearly half (49%) of the budget was allocated to research staff salaries and equity, highlighting the importance of talent in AI development. The rest was spent on specialized hardware (23%), server components (15%), and operational expenses (13%). This allocation underscores the complexity of AI development, which extends beyond just computing power to include human expertise and infrastructure.
Just eight years ago, training the original Transformer model cost $670, a stark contrast to today’s multi-million-dollar investments. Leading companies like Anthropic are now committing over $1 billion to train their models, while newer players such as DeepSeek-V3 claim to achieve similar results for as little as $6 million. While these figures vary, it’s clear that AI training costs have escalated dramatically, reshaping the market.
Implications for the market: centralization and competitive risk
As powerful AI tools become concentrated in the hands of a few large technology companies, other industries—like healthcare, finance, media, and retail could fall behind. These big tech firms have the resources to build or access advanced AI models, which allow them to work faster, make better decisions, and offer more personalized services. In contrast, smaller companies or those without access to such tools may struggle to compete, giving the tech giants a major advantage.
This growing gap risks consolidating AI innovation within a few dominant players, potentially limiting market diversity. Smaller companies may face difficulties in accessing cutting-edge AI capabilities, which could stifle their ability to innovate or remain competitive in the market.
Strategic takeaways for CEOs and business leaders
For business leaders, the increasing costs of AI training necessitate a strategic shift. Developing proprietary AI models may no longer be feasible for many organizations. Instead, partnerships, licensing agreements, or API integrations with larger companies that offer AI models could be more viable alternatives. Additionally, talent acquisition especially of top AI researchers has become just as critical as infrastructure investment.
The economics of AI development are transforming the business landscape. While the costs of AI models rise, the firms with the resources to innovate will shape the future. For most businesses, the focus should shift from developing new models to implementing existing models effectively and responsibly. Access to AI is becoming a key factor in determining who leads and who lags in the market