The Power of Demand Modeling for Optimized Pricing
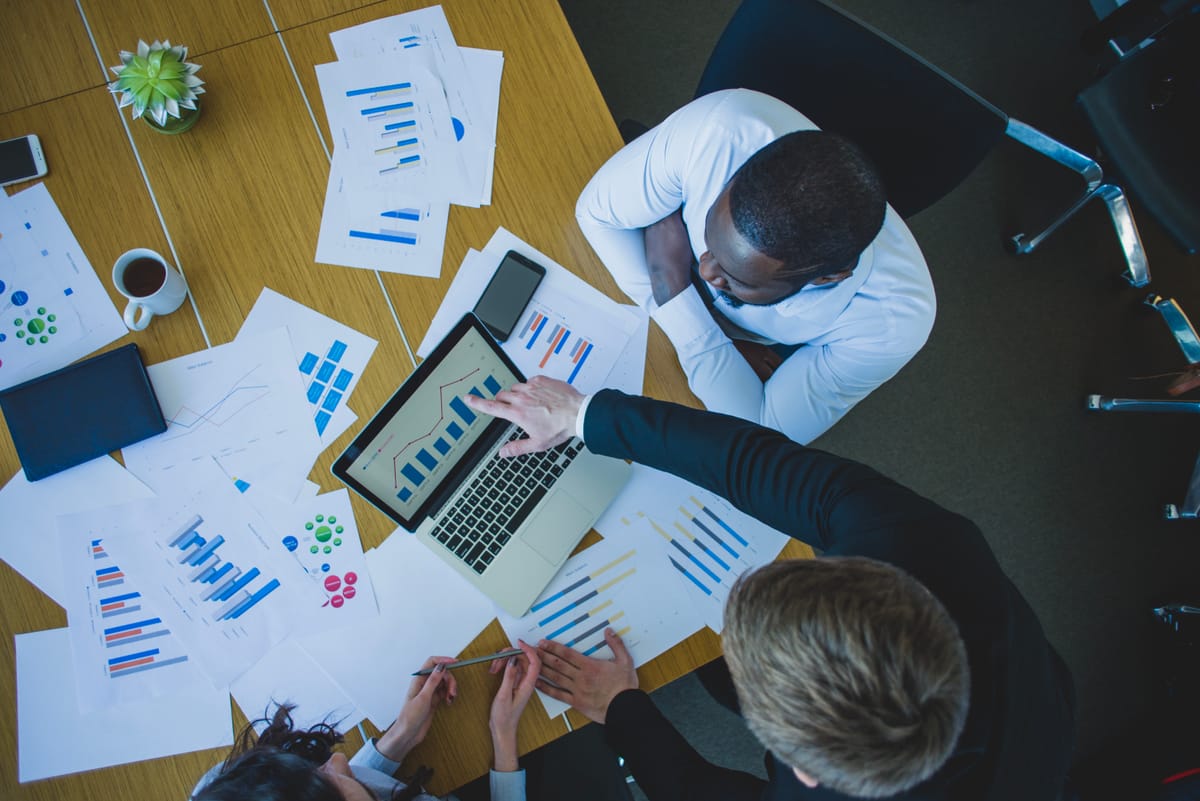
Finding the optimal price point to maximize revenues while maintaining volume is a persistent challenge. But advanced demand modeling techniques are empowering brands to make pricing decisions with scientific precision.
The Complexities of Pricing
Pricing a product or service involves balancing complex trade-offs between profit margins and sales volume. Price too high, and you risk suppressing demand. But price too low, and you leave profits on the table.
Traditionally, brands rely on intuitive trial-and-error to find optimal pricing. However, this approach is imprecise and time-consuming. Even small pricing missteps can significantly impact the bottom line. Just a 5% pricing adjustment can swing profits by 22% or more.
Given these high stakes, pricing deserves to be treated as a science, rather than an art.
Building Predictive Demand Models
Predictive analytics can estimate demand responses to price changes with greater accuracy than rules of thumb. By applying regression analysis and machine learning algorithms to historical sales data, demand modeling uncovers complex correlations between pricing and volume.
Key inputs for building demand models include:
- Past pricing tiers and unit sales across time periods
- Competitor pricing strategies and product positioning
- Consumer price elasticity, thresholds, and willingness to pay
- Seasonality, promotions, discounts, and external market factors
Armed with data-driven demand forecasts at granular price points, brands can optimize pricing to precisely meet goals, whether driving maximum revenues, margins, or market share.
Ongoing Optimization and Validation
The power of demand modeling is continuous optimization as new sales data is incorporated. By feeding model outcomes back into algorithms as an input, pricing strategies evolve and improve over time through machine learning.
Multivariate testing also allows validating model accuracy. Brands can A/B test prices in the real world while controlling other variables, comparing actual sales lift against modeled forecasts. This refines the models.
Applications Across Industries
Predictive demand modelling has applications across verticals like:
- Setting introductory prices for new products and services
- Planning promotional discounts and clearance sales
- Personalizing pricing with customer segment tiering
- Geographical pricing optimization and localization
- Adjusting channel margins for distributors and retailers
- Forecasting subscription renewals and contract renegotiations
The Future of Strategic Pricing
In today's hypercompetitive omnichannel environment, data-driven pricing using demand modeling reduces risk and unlocks significant value. The future of strategic pricing resides at the intersection of predictive analytics, optimisation engines and real-world validation. Leading brands are already pioneering demand modeling to power pricing advantage. Will you ride the wave or be swept away by the tidal forces of pricing innovation? The choice is yours.